A blog of the Science and Technology Innovation Program
According to a 2022 Pew Research Center study, 45% of Americans are both equally excited and concerned about the future of artificial intelligence (AI). Part of these concerns over AI stem from a fear and distrust of the unknown, as illustrated by a Forbes report that shows 80% of the surveyed businesses are hesitant to implement AI due to a lack of trust. Trust in AI applications has become a frequently discussed issue by both private and public sectors; in order to address the issue of trust, we must also consider another fundamental concern around AI: explainability.
This is particularly true in “black box” AI: a term used to describe AI models where you know what data goes in, and what comes out, but not how it got from point A to B. Even the engineers do not fully understand how their own models think and process information. Without any insights into how black box AI makes decisions, naturally, it becomes difficult to trust the results from these models. One field where this is evident, and where this technology is being applied in both research and practice, is the medical field.
Black Box AI in Medicine
Black box AI models became a popular tool in the medical field because of their superior speed and accuracy in analyzing large quantities of data. Medical AI applications have promised to revolutionize the healthcare industry, from drug discovery and diagnosis to management and patient treatment. Successes in the field have been found within applications such as the AlphaFold machine that predicted more than 200 million protein structures.
However, the medical field is extremely high-stakes and trust-based, and the opaqueness of black box AI has proven to be problematic. Our lack of understanding of black box AI’s inner workings has prohibited clinicians from interpreting and explaining model results. Since neither patients nor clinicians can ask questions about the decisions made by these technologies, it becomes very difficult to trust these AI models as shown by a study conducted by European researchers from Romania and the United Kingdom.
For instance, according to a study from Delft University in the Netherlands, IBM’s high-profile Watson for Oncology program failed largely because it could not provide any rationale for its diagnoses when they differed from the ones given by the doctors. Without any explanations, doctors refused to believe Watson’s recommendations were superior to their own, which were reason- and experience-based. Although training inaccuracy might also have contributed to Watson’s failure, almost all medical AI programs suffer from issues of distrust due to their limitations in explainability and justifiability.
The black box issue with AI is not just limited to the medical field. A June 2020 International Data Corporation report showed that 43% of business leaders believe explainability is an important factor in deploying AI. In order to allow AI to reach its full potential, we need to either open up the so-called the black boxes or develop other methods to ensure responsible AI development to cultivate trust.
Technical Solution: Explainable AI
As more black box models are being created and implemented, there is a growing need for stakeholders to better understand the thought process of these models, which has led to the rise of explainable AI, or XAI. Research in the XAI field focuses on both building transparent models by design and post-hoc explainability models. For the purpose of this blog, the focus will be on post-hoc explanations, which is currently the more widely-used method.
Post-hoc explainability is a set of processes that attempts to analyze and explain the outputs of pre-existing black box models. For example, given a black box model that classifies pictures of dogs and cats, a visual post-hoc explainability model can highlight which specific parts of the picture were used by the model to make its decision. In this case, ideally, the face or the body of the animal will be highlighted as the explaining factor. If the background is highlighted instead, we can then identify inaccuracies in the model before it is implemented.
Post-hoc explainability has found success in research and industry. Within the medical field, researchers at the University of Paris developed a way to better understand breast cancer through XAI. Multiple industries, such as banking and insurance, have also shown interest in incorporating XAI into their predictive algorithms.
However, using XAI alone is not yet enough to build trust in black box AI applications. A 2021 University College Dublin study showed that post-hoc explainability models can have very high and variable error-rates that can undermine trust in XAI algorithms, a concern shared by AI researchers around the world. Moreover, people might also misinterpret results of XAI models and assume non-existing causal relationships. In other words, just because A explains the actions of B does not mean A itself caused B to predict in a certain way. ProPublica’s well-known report on the racial bias within the COMPAS system, a landmark case in algorithmic bias, was even criticized by several AI researchers for assuming dependency between race and risk-scores and falling into this exact trap.
In the end, XAI is still a nascent field, and we cannot expect all black box models to be correctly explained, or explained at all, in the near future. But the issue of explainability alone will not slow the rapid development and deployment of AI in the public and private sectors. Therefore, AI decision-makers must identify alternative methods to build trust in black box AI without necessarily uncovering the black box.
Beyond Explainability: Transparent AI
Explainability alone is often not enough to foster trust in AI systems since humans inherently find it difficult to trust machines, for reasons such as model inaccuracies, algorithmic biases, and model misuses. Luckily, explaining black box models is not the only way to build trust. An alternative solution lies within developing AI users’ trust in the human creators behind black box AI models, which can be achieved by providing transparency in AI.
The Harvard Business Review calls transparency a “systematic transference of knowledge from one stakeholder to another." Farther reaching than AI explainability, transparency in AI refers to the ability to disclose information throughout an algorithm’s life cycle that is not limited to the model’s decision-making process. A truly transparent AI model requires clarity to be shown in many aspects of an AI model from design and training, to monitoring and validation, and to conditions of termination and beyond. Even though the public will not understand the algorithm itself, they can still trust it if they have faith that the model is developed and monitored responsibly and produces accurate and reliable outputs, such as through computational reliabilism.
Achieving AI transparency is definitely easier said than done, and it is totally up to the AI decision-makers to accomplish. Looking back to the Harvard Business Review's definition, transparency can be a difficult balancing act for companies with proprietary information and technologies. But even sharing the simplest information such as how data is used and where the model will be implemented can build trust by demonstrating a willingness to help people understand. More thorough AI transparency practices can also help identify potential sources of error and biases in models, which is another area correlated to public anxiety and distrust in AI systems.
Transparency and explainability are goals that policymakers, researchers, and AI decision-makers all need to strive towards as AI continues to play a larger role in the world. Yet, the current AI decision-making process is as much of a black box as the algorithms themselves even though there are no technological limitations preventing us from acquiring more transparency. Policymakers and the public need to hold AI decision-makers, namely private tech companies and governmental agencies, accountable for providing transparent information throughout their AI development process and researching and building more explainable models. Only then can we enjoy the fruition of these tools with trust and a peace-of-mind.
Author
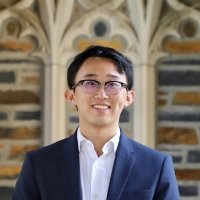
Science and Technology Innovation Program
The Science and Technology Innovation Program (STIP) serves as the bridge between technologists, policymakers, industry, and global stakeholders. Read more